Studies show that companies using predictive analytics for marketing decisions see up to 25% improvement in ROI compared to those relying on traditional forecasting methods. This powerful combination of historical data analysis and machine learning enables marketers to make more informed decisions about future investments and strategy adjustments.
Advanced analytics and big data have created new possibilities for understanding and predicting marketing performance with greater precision than ever before. Keep reading to learn how predictive analytics can enhance your approach to ROI forecasting and campaign optimization.
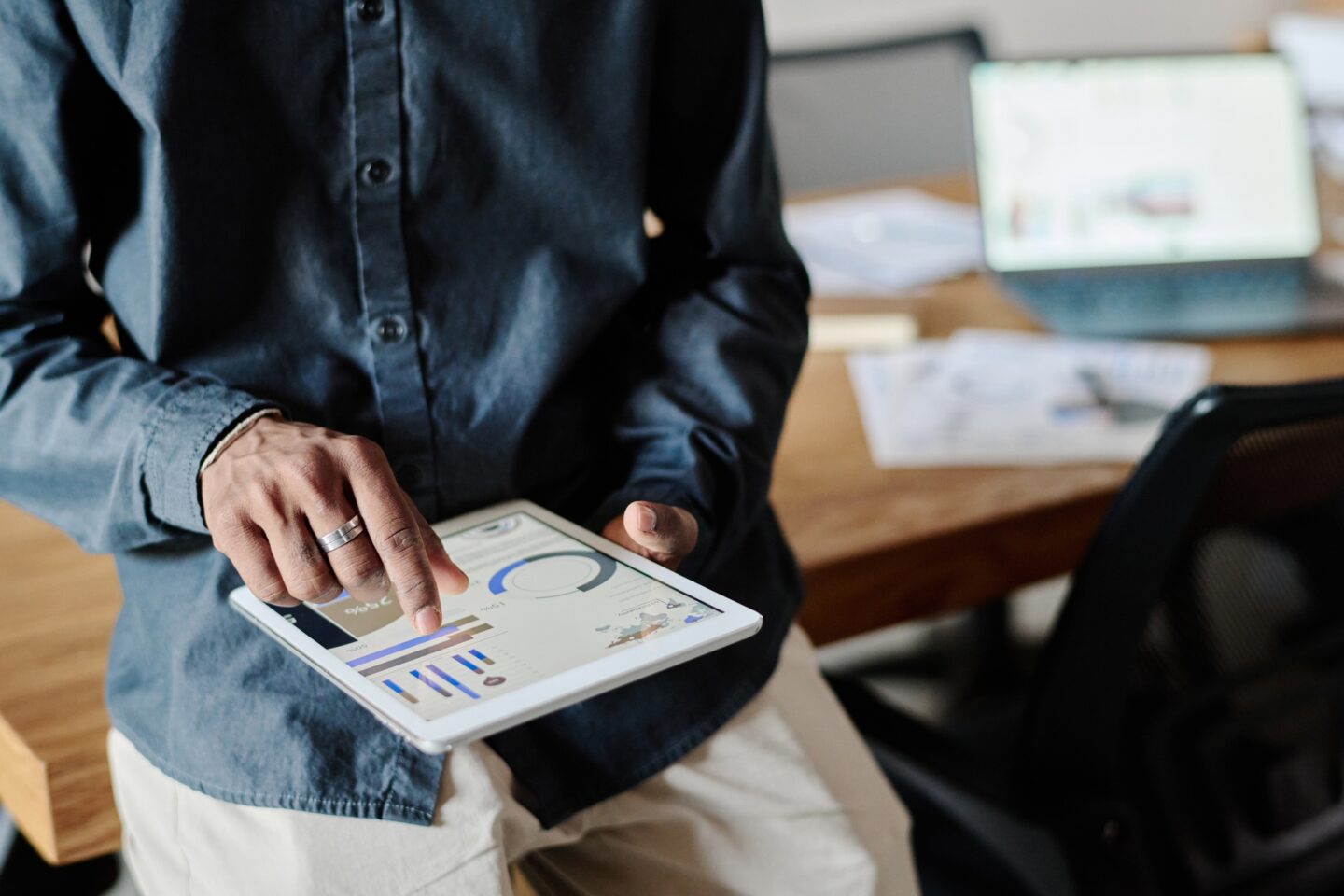
Understanding Predictive Analytics in Marketing
Predictive analytics in marketing combines historical data, statistical algorithms, and machine learning techniques to identify the likelihood of future outcomes. This approach moves beyond traditional marketing analytics by not only explaining past performance but also providing reliable forecasts about future opportunities.
For example, a major retail brand recently used predictive analytics to optimize its holiday campaign spending, resulting in a 20% increase in conversion rates and a 15% reduction in customer acquisition costs. This deeper level of insight allows for more precise budget allocation, improved targeting, and better-informed strategic decisions.
Key Components of Marketing Predictive Analytics
Marketing predictive analytics relies on diverse data sources to create accurate forecasting models. Popular tools like Google Analytics, Adobe Analytics, and Salesforce provide essential data streams, including customer relationship management (CRM) data, website analytics, social media metrics, and transaction records. The quality of these data sources directly impacts the accuracy of predictive models and their resulting forecasts.
Data preparation involves collecting both structured data, such as sales figures and click-through rates, and unstructured data, including customer reviews and social media interactions. These various data points work together to create a comprehensive view of marketing performance and potential future outcomes.
Common Predictive Modeling Techniques
Regression analysis serves as a fundamental technique in marketing ROI forecasting. For instance, an e-commerce company might use regression analysis to understand how advertising spend across different channels affects sales revenue, helping identify which platforms consistently deliver the highest returns.
Machine learning algorithms, particularly those focusing on pattern recognition and anomaly detection, offer more sophisticated approaches to ROI prediction. A subscription-based service might use these algorithms to predict customer churn rates and optimize retention marketing campaigns.
Time series analysis provides valuable insights into seasonal trends and cyclical patterns in marketing performance. Retail brands often use this technique to forecast peak shopping periods and adjust their advertising budgets accordingly.
Building a ROI Forecasting Framework
Creating a robust ROI forecasting framework requires careful planning and a systematic approach to data analysis. This foundation ensures that predictions remain reliable and actionable while adapting to changing market conditions and business needs.
Data Collection and Preparation
The first step in building a reliable forecasting system involves gathering clean, relevant data from all available marketing channels. Tools like Tableau, Power BI, and Python libraries such as Pandas help streamline this process, making it easier to identify key data sources, establish collection procedures, and implement quality control measures.
Data preparation involves cleaning and normalizing information to ensure consistency across different sources. This includes removing duplicates, handling missing values, and standardizing formats to create a unified dataset that can be effectively analyzed.
Model Selection and Development
Choosing the right predictive model depends on various factors, including the type of marketing activities being analyzed, the available data, and the specific forecasting goals. Simple linear models might work well for straightforward campaign analysis, while complex machine learning algorithms may be necessary for multi-channel attribution and long-term forecasting.
The development process involves testing different modeling approaches and selecting the one that provides the most accurate and reliable predictions for your specific use case. This includes considering factors such as model complexity, interpretability, and computational requirements.
Common Challenges and Solutions
Implementing predictive analytics often presents several key challenges that organizations must address. Data quality issues can be mitigated through robust validation processes and regular auditing procedures. Resource constraints can be managed by starting with smaller pilot projects and scaling gradually.
Securing organizational buy-in requires demonstrating clear ROI and maintaining transparent communication about both successes and limitations. Regular stakeholder updates and clear documentation of wins help build confidence in the predictive analytics program.
Transform Your Marketing ROI with Advanced Analytics
Predictive analytics has revolutionized marketing ROI forecasting, enabling organizations to make data-driven decisions with unprecedented accuracy. By implementing robust forecasting frameworks and maintaining a commitment to continuous improvement, companies can achieve up to 15-25% improvement in marketing performance and competitive advantage.
Ready to transform your marketing ROI with advanced analytics? Mynt Agency's team of experts combines deep media buying experience with cutting-edge predictive analytics capabilities to optimize your marketing investments. Contact us today to discover how our data-driven approach can help you achieve more precise forecasting and superior campaign performance.