According to Gartner, organizations with integrated data systems see a 20-30% increase in marketing ROI compared to those with siloed data. This stark difference highlights the critical impact of data fragmentation on marketing effectiveness in today's digital landscape, where brands interact with customers across numerous touchpoints.
As marketing technology stacks continue to expand, the challenge of data fragmentation becomes more pressing. Keep reading to learn how breaking down data silos can transform your attribution modeling and provide a clearer picture of marketing performance.
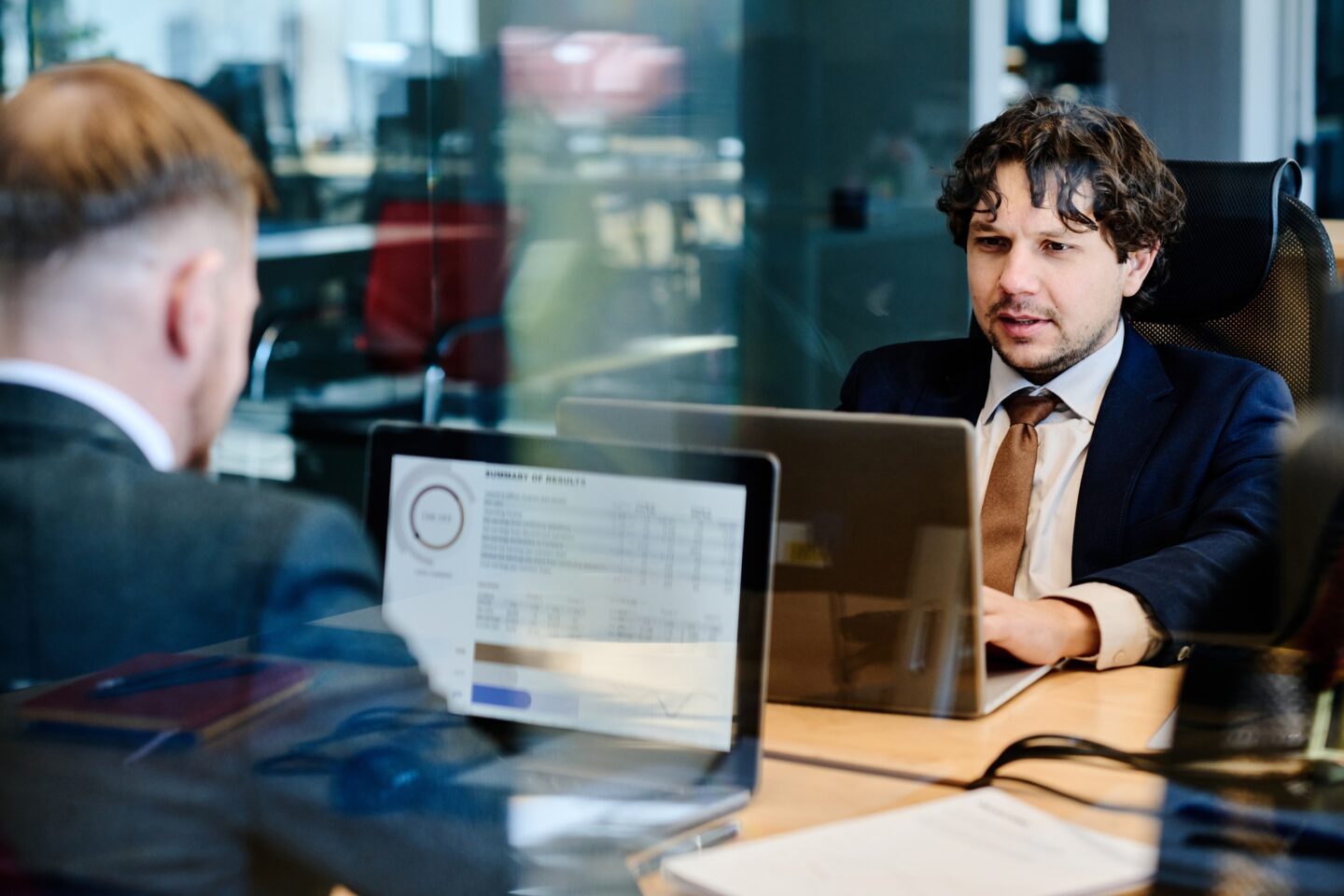
Understanding Data Silos in Marketing Attribution
Data silos occur when information is stored in separate systems that don't effectively communicate with each other. In marketing organizations, these silos typically form as teams adopt specialized tools and platforms to manage different aspects of their campaigns, creating blind spots in attribution modeling and making it difficult to track the complete customer journey.
Marketing teams commonly use various platforms like Google Analytics, Facebook Ads Manager, and Salesforce CRM to manage different aspects of their campaigns. While each platform excels at its specific function, they often operate independently, creating gaps in data collection and analysis.
Common Types of Marketing Data Silos
Platform-specific silos are perhaps the most prevalent in marketing organizations. Social media platforms like Facebook, Instagram, and LinkedIn each maintain their own data ecosystems, making it challenging to connect user interactions across these channels. Similarly, advertising platforms like Google Ads and programmatic networks often operate in isolation, leading to incomplete views of campaign performance.
Departmental silos emerge when different teams within an organization use separate tools and maintain their own data sets. For example, the social media team might use one set of analytics tools while the paid advertising team uses another. This separation can lead to inconsistent reporting and difficulties in understanding how different marketing efforts work together.
Technical silos arise from incompatible data formats, different tracking methodologies, and varying attribution windows across platforms. These technical barriers can make it particularly challenging to create a unified view of marketing performance, even when teams are actively trying to share data.
The Cost of Fragmented Attribution Data
Organizations with fragmented data systems often experience significant waste in their marketing budget due to poor attribution. When data exists in silos, marketers frequently miss important conversion touchpoints or double-count others, resulting in skewed ROI calculations and inefficient budget allocation.
Beyond direct financial costs, siloed data prevents marketers from developing a comprehensive understanding of the customer journey. This limitation affects strategic decision-making, campaign optimization, and the ability to deliver personalized experiences that modern consumers expect.
Technical Solutions for Breaking Down Data Silos
Modern technology offers several approaches to integrate disparate data sources and create a unified view of marketing performance. These solutions range from basic API integrations to sophisticated customer data platforms, each offering different levels of complexity and capability.
API Integration Strategies
API integrations serve as the foundation for connecting different marketing platforms and creating a seamless flow of data. Common implementations include Google Analytics API integration with Salesforce Marketing Cloud and Facebook Ads API connection with CRM systems.
Webhooks enable real-time data synchronization between platforms, ensuring that attribution models remain current and accurate. When a conversion occurs in one system, webhooks can automatically update related systems, maintaining data consistency across the marketing stack.
Custom API solutions offer flexibility when native integrations don't meet specific needs. These solutions can be tailored to accommodate unique business requirements and complex attribution models, though they require more technical resources to implement and maintain.
Data Warehouse Implementation
Data warehouses provide a centralized repository for marketing data from multiple sources. By consolidating data in a single location, organizations can create a consistent view of marketing performance and enable more sophisticated attribution modeling.
Solutions like Snowflake, BigQuery, and Amazon Redshift offer scalable options ranging from $1,000 to $10,000 per month, depending on data volume and processing needs. These systems can handle large volumes of historical data while maintaining fast query performance, making them ideal for complex attribution analysis.
Customer Data Platforms (CDPs)
Customer Data Platforms specialize in creating unified customer profiles by collecting and connecting data from multiple sources. They excel at identifying individual users across different channels and devices, making them particularly valuable for attribution modeling in today's multi-channel marketing environment.
Popular CDP platforms include Segment, Tealium, and mParticle, each offering unique features for data collection, identity resolution, and activation. Implementation costs typically range from $50,000 to $150,000 annually, with enterprise solutions commanding higher prices.
For mid-sized businesses, CDPs can deliver ROI through improved targeting accuracy and reduced media waste. Enterprise organizations often see additional benefits through advanced segmentation and real-time personalization capabilities.
Best Practices for Unified Attribution Modeling
Creating a unified attribution system requires careful planning and ongoing maintenance. Success depends on establishing clear processes and following best practices for data management and integration.
Data Governance Framework
A robust data governance framework ensures consistency and reliability in attribution modeling. This framework should include clear guidelines for data collection, standardization of naming conventions, and regular auditing procedures to maintain data quality.
Organizations should establish clear ownership of data governance and create documentation that guides teams in maintaining consistent data practices. Regular reviews of data collection methods and attribution rules help maintain the integrity of the unified system.
Change Management and Team Training
Successful implementation of unified attribution systems requires strong change management practices and comprehensive team training. Organizations should develop training programs that cover both technical aspects and strategic implications of the new attribution approach.
Regular workshops and knowledge-sharing sessions help maintain alignment across teams and ensure consistent data practices. Creating clear documentation and establishing support channels can help teams adapt to new processes more effectively.
Cross-Channel Tracking Implementation
Implementing consistent tracking across marketing channels starts with establishing standardized parameters and conventions. UTM parameters should follow a uniform structure across all campaigns, making it easier to connect data points and attribute conversions accurately.
The implementation process should include mapping customer journeys to identify all potential touchpoints and ensuring proper tracking is in place for each interaction. This comprehensive approach helps create a more accurate attribution model.
Quality Assurance and Validation
Regular testing and validation procedures help maintain the accuracy of unified attribution systems. Teams should establish protocols for checking data consistency across platforms and verifying that integrations are functioning correctly.
Data validation methods should include automated checks for common issues like duplicate entries, missing values, and incorrect formatting. Regular audits help identify and resolve problems before they significantly impact attribution accuracy.
Future-Proofing Your Attribution Model
The landscape of marketing attribution continues to evolve with new privacy regulations and technological changes. Organizations can prepare for these changes by implementing server-side tracking solutions and developing robust first-party data collection strategies.
Privacy-compliant measurement approaches, such as data clean rooms and privacy-preserving APIs, are becoming essential tools for future-ready attribution systems. Machine learning and AI continue to advance, offering new possibilities for processing complex attribution data and identifying patterns in customer behavior.
Transform Your Marketing Attribution Today
Breaking down data silos is no longer optional in today's complex digital landscape. Organizations that successfully integrate their marketing data can achieve up to 30% better campaign performance and make significantly more informed decisions about budget allocation and strategy.
Mynt Agency's expertise in cross-channel campaign management and attribution modeling can help you navigate these challenges. Our team specializes in implementing comprehensive attribution solutions that connect data across all marketing channels while maintaining privacy compliance and future readiness. Contact us today to discover how we can transform your marketing attribution and maximize your campaign effectiveness.